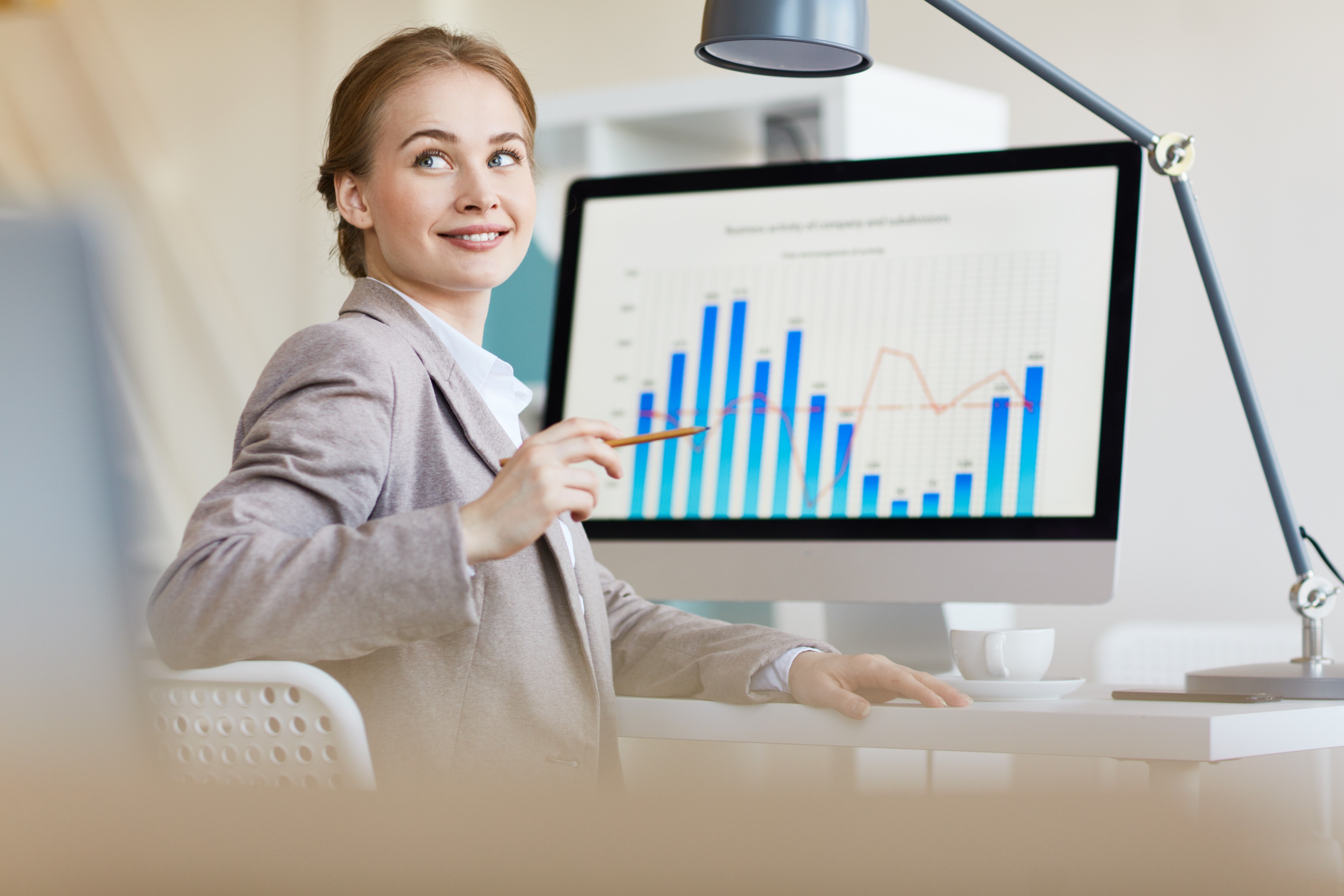
Know how advanced analytics in healthcare Improving health outcomes?
Advanced analytics in healthcare refers to the use of sophisticated analytical techniques and tools to derive insights from vast amounts of data in order to improve patient outcomes, streamline operations, and reduce costs. The importance of advanced analytics in healthcare cannot be overstated, as it has the potential to transform the way healthcare is delivered, leading to better patient outcomes, more efficient use of resources, and a more personalized approach to care.
Big Data Analytics in Healthcare:
Big data analytics involves the analysis of large and complex data sets to identify patterns, trends, and insights that would be difficult to identify through traditional data analysis methods. In healthcare, big data analytics is being used to improve patient outcomes and reduce costs. By analyzing large data sets, healthcare providers can identify patterns in patient behavior, treatment outcomes, and disease progression.
One example of big data analytics in healthcare is the use of electronic health records (EHRs). EHRs contain a wealth of information about a patient's medical history, including diagnoses, treatments, medications, and lab results. By analyzing this data, healthcare providers can identify trends and patterns in patient outcomes, identify high-risk patients, and develop targeted treatment plans.
Another example of big data analytics in healthcare is the use of real-time monitoring. Real-time monitoring involves using sensors and other devices to collect data on a patient's vital signs and other health metrics. This data is then analyzed in real-time to identify potential health risks and alert healthcare providers if intervention is needed.
Predictive Analytics in Healthcare:
Predictive analytics involves using historical data to predict future outcomes. In healthcare, predictive analytics is being used to identify patients who are at high risk for certain conditions, such as heart disease, diabetes, and cancer. By identifying high-risk patients, healthcare providers can develop targeted interventions to prevent or manage these conditions.
The primary function of predictive analytics in healthcare is the use of risk models to identify patients who are at high risk for readmission to the hospital. By analyzing a patient's medical history and other factors, such as age and comorbidities, healthcare providers can identify patients who are at high risk for readmission and develop interventions to prevent it.
Predictive analytics in healthcare also grants importance to the use of machine learning algorithms to foresee patient outcomes. By analyzing large data sets, machine learning algorithms can identify patterns and predict patient outcomes with a high degree of accuracy. This information can be used to develop targeted treatment plans and improve patient outcomes.
Population Health Analytics:
Population health analytics involves the analysis of data from large groups of people to identify trends and patterns in health outcomes. In healthcare, population health analytics is being used to identify health disparities and develop targeted interventions to improve health outcomes for specific populations.
An example of population health analytics in healthcare is the use of data from public health surveys to identify health disparities. By analyzing data from public health surveys, healthcare providers can identify populations that are at higher risk for certain conditions, such as obesity and diabetes. This information can be used to develop targeted interventions to improve health outcomes for these populations.
Another way by which population health analytics strengthens healthcare is by the use of geographic information systems (GIS) to identify health disparities. By analyzing data on health outcomes and socioeconomic factors by geographic location, healthcare providers can identify areas with high rates of certain conditions and develop interventions to improve health outcomes in these areas.
Business Analytics in Healthcare:
Business analytics involves the analysis of data related to the operations and financial performance of an organization. In healthcare, business analytics is being used to optimize costs and improve efficiency.
The use of data on patient flow to optimize staffing levels is a critical aspect of it. By analyzing data on patient volume and flow, healthcare providers can adjust staffing levels to ensure that resources are used efficiently. Along with that, business analytics in healthcare is important for it betters the use of predictive modeling to forecast future demand for healthcare services
Advanced Analytics in Healthcare Faqs:
1. What are examples of advanced analytics?
Examples of advanced analytics include machine learning, predictive analytics, data mining, natural language processing, and deep learning.
2. What are examples of analytics used in healthcare?
Analytics used in healthcare include population health management, clinical decision support, patient engagement, disease surveillance, and quality improvement.
3. What are the 4 types of healthcare data analytics?
The four types of healthcare data analytics are descriptive analytics, diagnostic analytics, predictive analytics, and prescriptive analytics.
4. What are the 4 P's of health analytics?
The 4 P's of health analytics are Patients, Providers, Payers, and Policy-makers.
5. What are the most important applications of data analytics in healthcare?
The most important applications of data analytics in healthcare include improving patient outcomes, reducing healthcare costs, increasing operational efficiency, enhancing population health, and improving the quality of care.